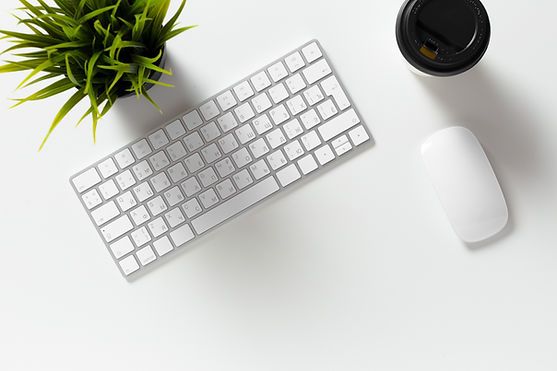
Unsupervised Machine Learning for Analysis of Young Star Photometric Light Curves
Summer Undergraduate Research Fellowship at Caltech (2024)
Time series data such as stock market prices in finance or light curves in astronomy present a notoriously challenging problem in machine learning. Spectral clustering is a type of unsupervised machine learning that transforms data points into a graph structure where each point is a node and edges represent the similarity between nodes. I apply spectral clustering to the normalized flux of light curves in the Upper Scorpius and Taurus clusters in the K2 and TESS datasets to obtain a nuanced understanding of stellar variability and characteristics beyond those available using standard light curve metrics. I utilize quantile graphs for dimensionality reduction, capturing the general motion of the light curves while remaining robust to attributes such as amplitudes, period, and phase as well as fluctuations in the data. By applying an unsupervised method to a traditionally supervised task, I aim to uncover novel insights in the light curves.​​
TOOLS
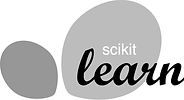
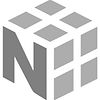

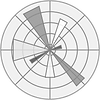